AI: ensuring GDPR compliance
Artificial intelligence raises crucial and new questions, especially with regard to data protection. Here, the CNIL provides a reminder of the main principles of the French Data Protection Act and the GDPR to be followed, along with its position on certain more specific aspects.
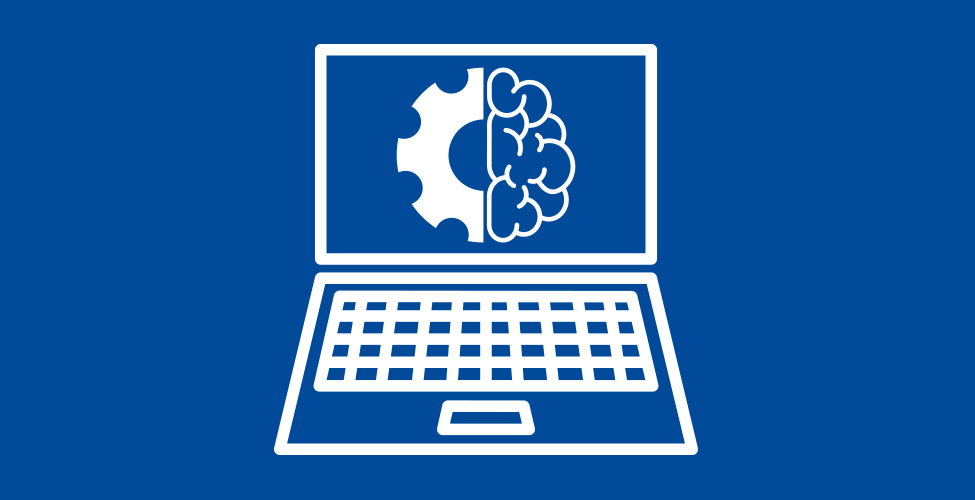
Defining a purpose
Principle
In order to comply with the GDPR, an artificial intelligence (AI) system based on the use of personal data must always be developed, trained, and deployed with a clearly-defined purpose (objective).
This objective must be determined, in other words established in advance at the design stage of the project. It must also be legitimate, and therefore compatible with the organisation's missions. Lastly, it must be clear, in other words known and understandable.
In practice
As with all data processing, but all the more so when very large amounts of personal data are involved – as is often the case with AI systems – respect for this principle must be ensured.
This is especially important as it is the purpose that ensures that only relevant data is used and that the retention period is appropriate.
Learning vs production: the specific case of AI systems
The implementation of an AI system based on machine learning requires two successive phases:
-
Learning phase
The learning phase involves designing, developing and training an AI system and in particular a model, in other words a representation of what the AI system will have learned from the training data.
-
Production phase
The production phase consists of the operational deployment of the AI system obtained in step 1.
In terms of data protection, these two steps do not serve the same objective and should therefore be separated.
In both cases, the purpose of the personal data processing carried out during each phase must be determined, legitimate and clear.
Establishing a legal base
Principle
As with all processing, an AI system using personal data can only be implemented for a use justified by the law. The GDPR sets out 6 such legal grounds: consent, compliance with a legal obligation, performance of a contract, completion of a public interest mission, the safeguarding of vital interests and the pursuit of a legitimate interest. More specifically, the legal basis is what gives an organisation the right to process personal data. Choosing this legal basis is therefore an essential first step in ensuring compliant
processing. Depending on the base chosen, the obligations of the organisation and the rights of the individuals may vary.
The legal basis must be chosen prior to the implementation of the data processing.
Although fundamentally there is no difference between the implementation of an AI system and any other processing of personal data, there are some specific aspects that require vigilance. AI systems – and in particular those based on machine learning – therefore need to use data in the learning phase before being applied to other data in the operational phase.
In any case, an AI system cannot be implemented on personal data collected illegally, whether in the learning phase or the operational phase. Further details can be found in the following section, “Compiling a database”.
Furthermore, where data has been collected under another regime (such as the Law Enforcement Directive for example), the processing of personal data for learning purposes is, except in specific cases, covered by the GDPR provided that:
- this learning phase is clearly separate from the operational implementation of the AI system (see the “Learning vs production: the specific case of AI systems” box in the previous section “Defining a purpose”);
- its exclusive purpose is to develop or improve the performances of an AI system.
Warning: the objective of “scientific research” cannot in itself constitute a legal basis for processing. Only the legal basis listed in the GDPR can allow personal data to be processed.
Compiling a database
AI systems, and in particular those based on machine learning, require large volumes of data. These are essential, both for the training of systems and for assessment, benchmarking and validation purposes. The constitution of datasets has always been a challenge for computer science and requires a major effort, since they must be combined with annotations describing the data and be labelled, cleaned, standardised, etc. It is therefore an essential challenge in artificial intelligence processing.
In practice
There are two main options for the constitution of datasets: the specific collection of personal data for this purpose and the re-use of data already collected for another purpose. In the latter case, the question arises as to whether the purposes for which the data was initially collected are compatible with the conditions under which the initial dataset has been constituted.
In any case, the constitution of datasets containing personal data, often based on long data retention periods, must not be to the detriment of the rights of the data subjects. In particular, it should be accompanied by information:
- either prior to the data collection;
- or within one month after the reception of the datasets by the third parties.
This information is essential for the exercise of other rights (access, rectification, erasure, objection).
Examples
- In the field of health, the CNIL has had the opportunity to give its opinion on the creation of health data warehouses. In recently published guidelines, it specifies the framework within which data can be collected and retained in a single database for a long period of time, as part of public interest missions and for subsequent research.
- In the context of a request for advice, the CNIL services were able to accept the re-use of video protection images in a particular context for scientific research on understanding crowd movements, a task in the field of computer vision. However, it was specified that in order to do so, the collection should:
- take place within the legal retention period for video protection images (1 month); and
- be accompanied by information for the data subjects.
Minimising data
Principle
The personal data collected and used must be appropriate, relevant and limited to what is necessary for the defined objective: this is the principle of data minimisation. Great attention must be paid to the nature of the data and this principle must be applied particularly rigorously when the data processed is sensitive data (Article 9 of the GDPR).
The most prominent and discussed AI systems today are based on extremely powerful machine learning methods. The improvement of these methods has been made possible by the combined effects of:
- the research and development of new approaches;
- the increase in computing power available to perform more complex operations; and
- the increasing volumes of data available.
While the use of large amounts of data is central to the development and use of AI systems, the minimisation principle is not in itself an obstacle to such processing.
In practice
It is necessary to determine the types of data needed to train and operate an AI system, for example by means of experiments and tests on fictitious data, in other words data with the same structure as real data but not linked to an individual. This data does not then constitute personal data.
The quantity of data needed to train the system must also be accurately estimated and balanced against the purpose of the processing, in line with the proportionality principle.
The learning (or training) phase effectively aims to develop an AI system and thus to explore the possibilities offered by machine learning, and may require a large amount of data, some of which will ultimately prove useless in the deployment phase.
Reasonable use must therefore be made of the data. In practice, it is thus recommended (and this list is non-exhaustive) to:
- critically assess the nature and quantity of data to be used;
- verify the performances of the system when supplied with new data;
- clearly distinguish between data used in the learning and production phases;
- use data pseudonymisation or filtering/masking mechanisms;
- establish and keep available documentation on how the dataset used is compiled and its properties (data source, data sampling, verification of its integrity, cleaning operations carried out, etc.);
- regularly reassess the risks for the data subjects (privacy, risk of discrimination/bias, etc.);
- ensure data security, and in particular provide a precise framework for access authorisations in order to limit the risks.
Exemple
As part of clinical research aimed at identifying explanatory variables for prostate cancer, the CNIL refused to allow a pharmaceutical laboratory to process data for the entire active patient population from the medical records of the various centres participating in the study.
This active patient population in fact contained hundreds of millions of records from individuals not suffering from prostate cancer (and even records for women!). The desire to process this data, which is scientifically explained by the need for “true negatives” in order to effectively train a classifier, did indeed appear to be disproportionate to the purpose of the processing, and not necessary for the development of an effective AI system.
Learning vs production - the specific case of AI systems
During the learning phase, relatively flexible supervision is possible with regard to access to sufficient volumes and sufficiently diverse data, subject to counterparts proportional to the risks raised by the processing (in particular, the nature of the data, its volume and the purpose of the AI system must be taken into account). The measures may include:
- access limited to a restricted number of authorised individuals;
- processing for a limited time, data pseudonymisation;
- the implementation of appropriate technical and organisational measures;
- etc.
Only after the learning phase has been completed can the deployment of the AI system to the production phase be considered. For this second phase, when leaving the “laboratory” environment, greater constraints will have to be implemented to monitor the processing.
For example, it will be necessary to narrow down the type of personal data to include only data that has proved essential following the learning phase and to determine appropriate measures, since production constraints differ from design and development constraints, so long as this first phase does not present particular risks for individuals.
Examples
- As part of a project submitted by an administration, the CNIL had the opportunity to rule on the difference between the learning (or development) phase and the operational (or production) phase of an AI system. In this project, the first (learning) phase was intended to be authorised by decree. If this phase had proved satisfactory, a second decree would then have been issued to regulate the practical implementation of this reference framework for professionals and the general public.
- * In the field of health, a clear distinction is made between the research phases, which require formalities to be completed with the CNIL (authorisation, compliance with a reference methodology, etc.), and the phases of use in a care pathway, which do not require any formalities to be completed with the CNIL.
Defining a retention period
Principle
Personal data cannot be retained indefinitely. The GDPR requires a time limit to be specified beyond which data must be deleted, or in some cases archived. This retention period must be determined by the data controller based on the objective for which the data was collected.
The implementation of an AI system may in many cases require the retention of personal data for a longer period of time than for other processing operations. This can be the case for the compilation of datasets for training and developing new systems, but also to meet the requirements of traceability and performance measurement over time when the system is put into production.
The need to define a retention period for the data does not prevent the implementation of AI processing operations. This period must always be proportional to the purpose: for example, the performance measurement purpose must be clearly scheduled in order to be used, and the data retained longer for this purpose must be selected appropriately. The simple purpose of measuring performance over time is not, a priori, sufficient to justify the retention of all data for long periods.
However, for AI processing performed for the purposes of scientific research, the data can be retained for longer periods of time.
Supervising continuous improvement
The distinction between the learning and production phases is not always clear for all AI systems. This is particularly the case with “continuous” learning systems, where the data used during the production phase is also used to improve the system, thus creating a complete feedback loop. The relearning process can be considered at different frequencies, for example after a few hours, days or months, depending on the objective.
Questions to ask
Apart from the risks of drift inherent in continuous learning (introduction of discriminatory bias, deterioration of performances, etc.), such a use of data for two distinct purposes (that for which the AI system is put into production and the intrinsic improvement of the system) raises questions in terms of data protection:
- To what extent are these two purposes inseparable?
- Is it possible to separate the learning and production phases in all cases?
- If the algorithm is provided by a publisher and used by a third-party data controller, how should the liabilities for the two phases of processing be broken down?
Examples
- In the cases it has ruled on, the CNIL has always considered that it was possible to separate the learning and production phases, even if they were intertwined. For example, in its white paper on voice assistants, the CNIL analyses the scenario of the re-use of data collected by a voice assistant in order to improve the service. The example of the annotation of new learning examples to improve the performance of artificial intelligence systems is specifically mentioned and a clear distinction is made between this processing and that implemented for the execution of the service expected by the voice assistant user.
- With regard to the division of liabilities between the parties involved, the CNIL recently ruled on the question of re-use by a data processor of data entrusted to them by a data controller. Applied to the case of AI systems, re-use by a system provider is legally possible if several conditions are met: authorisation from the data controller, compatibility test, informing of individuals and respect for their rights, and compliance of the new processing implemented.
Safeguarding against the risks involved with AI models
Main risks
Machine learning is based on the creation of models. These are representations of what the AI systems have learned from the training data. Since around 2010, an important field of research has emerged on the subject of securing AI models and in particular the possibilities of information retrieval, which may have important implications for the confidentiality of personal data.
These are frequently referred to as membership inference attacks, model evasion attacks or even a model inversion (see the LINC article "Petite taxonomie des attaques des systèmes d’IA” (Small taxonomy of attacks on AI systems)).
For example, numerous studies have shown that large language models (GPT-3, BERT, XLM-R, etc.) tended to “memorise” certain textual elements on which they had been trained (surname, first name, address, telephone number, credit card number, etc.). The possibility of carrying out such attacks and retrieving information from them calls into question the very nature of these new objects introduced by artificial intelligence. Both technical and organisational measures must therefore be implemented to minimise the risks (see the LINC publications on the security of AI systems).
Furthermore, an AI model trained on personal data cannot, by default, be considered itself to constitute personal data (or more precisely a set of personal data). Its compilation must however be based on lawful use of the data within the terms of the GDPR. Some regulatory authorities have thus been able to demand the deletion of AI models built on the basis of illegally collected data (for example the Federal Trade Commission in the United States).
Finally, if an AI model is subject to a successful privacy attack (by membership inference, evasion or inversion, for example), this may constitute a data breach. The model in question must then be withdrawn as soon as possible and the data breach notified to the competent data protection authority if the breach is likely to result in a risk to the rights and freedoms of the data subjects.
Examples
The CNIL had the opportunity to discuss the status of AI models under the GDPR with various organisations. To date, the CNIL does not consider an AI model trained on personal data to necessarily contain personal data.
Nevertheless, since there are real risks of a breach of privacy rights, the CNIL recommends that appropriate measures be implemented to minimise them. Therefore, as part of the support provided for one of the winning personal data “sandbox” projects, the question of the nature of AI models learned locally and reported to an orchestrator centre when implementing federated learning methods was raised.
Providing information and explicability
Principle
The transparency principle of the GDPR requires any information or communication relating to the processing of personal data to be concise, transparent, understandable and easily accessible, using clear and plain language.
In practice
Although the main principles of the GDPR and the French Data Protection Act apply in the case of AI systems, the information to be given to individuals may vary:
- where the data has not been collected directly by the data controller implementing the AI system and it is difficult to get back to the data subjects. This problem is not specific to AI processing, but is frequently encountered here, particularly when using datasets in the learning phase;
- for the exercise of certain rights (in particular Article 22 of the GDPR), it is essential to provide precise explanations to the data subject on the reasons for the decision in question. The complexity and opacity of some AI systems can make it difficult to provide these elements.
In some cases, the right to be informed can be waived if the data has not been collected directly from the data subjects, in particular if it is demonstrated that informing the data subjects is impossible or requires disproportionate efforts, for example for AI processing carried out for scientific research purposes. In recent CNIL publications on the subject of scientific research (excluding health), one of the fact sheets specifically sets out the procedures for waiving the right for individuals to be informed.
Example
Following an inspection of a platform used to pre-register for the first year of a post-baccalaureate course, the CNIL found that there was a lack of information on the use of an algorithm and how it worked to rank and assign students to higher education establishments, which led to an order to the administration operating this platform.
This constituted a breach of Article 39.I.5 of the French Data Protection Act: “any natural person who can prove their identity has the right to question the data controller responsible for processing personal data in order to obtain: information enabling them to know and challenge the logic behind the automated processing in the event of a decision being taken on the basis thereof and producing legal effects with regard to the subject concerned”.
The CNIL therefore requested that the taking of decisions with legal effects for individuals solely on the grounds of automated data processing be stopped. In particular, a human intervention to take into account the observations of the individuals was requested.
Implementing the exercise of rights
Le principe
Data subjects possess rights to help them keep control of their data. The data controller of the file must explain to them how to exercise their rights (to whom? in what form?, etc.). When exercising their rights, individuals should, in principle, receive a response within one month.
Where the AI system involves the processing of personal data, it must be ensured that the principles for the exercising of rights by individuals under the GDPR are respected: access (Article 15), rectification (Article 16), erasure (Article 17), restriction (Article 18), portability (Article 20) and objection (Article 21). These rights offer essential protection for individuals, allowing them not to suffer the consequences of an automated system without having the possibility to understand and, if necessary, object to data processing that concerns them. In practice, these rights apply throughout the life cycle of the AI system and therefore cover personal data:
- contained in the datasets used for learning;
- processed in the production phase (which may include the outputs produced by the system).
Data controllers must therefore be aware from the system design stage that they must include appropriate mechanisms and procedures for responding to requests that may be received. Exceptions to the exercising of certain rights may be invoked in the case of AI processing carried out for scientific research purposes.
Furthermore, learned AI models are also likely to contain personal data:
- by construction, as is the case for certain specific algorithms that may contain fractions of training data (e.g. SVM or some clustering algorithms);
- by accident, as described in the section “Safeguarding against the risks involved with AI models”.
- par construction, comme c’est le cas pour certains algorithmes particuliers qui peuvent contenir des fractions de données d’apprentissage (par exemple SVM ou certains algorithmes de clustering) ;
- par accident, comme cela est indiqué dans la section « Se prémunir des risques liés aux modèles d’IA ».
In the first scenario, based on the technical possibilities available and the ability of the data controller to (re)identify the data subject, the exercising of their rights can be achieved.
In the latter scenario, it may be difficult or even impossible to exercise and comply with the rights of the data subjects.
The data controller must not collect or retain additional information to identify the data subject for the sole purpose of compliance with the GDPR (Article 11). In some cases, therefore, the identification of individuals can be complex. If the data controller demonstrates that it is unable to do so, then it may disregard the rights, without prejudice to individuals, to provide additional information, which may allow them to be re-identified in the processing. This will be the case for example when an individual feels that an AI system treats them in a particular way.
Complying with a request to correct or delete learning data does not therefore necessarily mean correcting or deleting the AI model(s) generated from this data.
Supervising automated decisions
Principle
Individuals have the right not to be subject to a fully automated decision (Article 22 of the GDPR) - often based on profiling - which has a legal effect or significantly affects them. However, an organisation can automate this type of decision if:
- the individual has given their explicit consent;
- the decision is necessary for a contract agreed with the organisation; or
- the automated decision is authorised by specific legal provisions.
In these scenarios, it must be possible for individuals to:
- be informed that a fully automated decision has been taken about them;
- request to know the logic and criteria applied to make the decision;
- challenge the decision and express their point of view;
- request human intervention to review the decision.
In practice
AI systems are often part of processing that can implement mechanisms making automated decisions.
The data controller must therefore anticipate the possibility of human intervention to enable the data subjects to have their situation reviewed, to express their point of view, to obtain an explanation of the decision made and to contest the decision. In the case of help with decision-making, guarantees are also needed, particularly in terms of information.
Examples
The question arises as to the definition of what constitutes an automated individual decision and the degree of human intervention that is desirable in the case of AI systems.
In its draft guide to recruitment, the CNIL analyses the use of certain automated tools to rank and even assess applications. Such solutions may lead to a “decision based exclusively on automated processing” by design when applications are rejected, or when applications are relegated to a secondary level not monitored by a human due to a lack of time, for example. Because of the risks associated with this method of decision-making, which is often opaque to candidates, such processes are in principle prohibited by the GDPR. Their use is allowed only in exceptional circumstances and is subject to the implementation of specific safeguards to protect the rights and interests of candidates.
The CNIL had the opportunity to issue an opinion on data processing implemented by an administration and aimed, on an experimental basis, at using content freely accessible online on platforms that put several parties in contact with each other with a view to selling a good, providing a service or exchanging or sharing a content, good or service. In this opinion, the CNIL specified that the data modelled by the processing operation should in no case lead to the automatic scheduling of tax audits nor, even more importantly, to decisions directly enforceable against taxpayers.
Assessing the system
Assessment angles
The assessment of AI systems is a key issue and at the heart of the European Commission's draft regulation. From a data protection perspective, this is essential for:
- Validating the approach tested during the system design and development phase (known as the “learning phase”). The aim is to verify as scientifically and honestly as possible that it works in accordance with the designers' expectations and, if necessary, is suitable for deployment in the production phase.
- Minimising the risks of system drift that can be observed over time. This could for example be because it is aimed at individuals with different profiles from those whose data makes up the dataset used in the learning phase, or because the system is regularly re-trained, which can lead to a deterioration in performance levels, potentially harmful to the data subjects.
- Ensuring that the system, once deployed in production, meets the operational requirements for which it was designed. The performances obtained during the learning phase must in fact be dissociated from those of the system once in place in the production phase, as the quality of the former is no indication of the quality of the latter.
Example
In a context of experimentation of facial recognition technology, the CNIL demanded that the report sent to it be accompanied by a thorough assessment protocol enabling the precise contribution of this technology to be measured. In practice, it asked to be provided with:
- objective performance metrics commonly used by the scientific community;
- a systematic analysis of system errors and their operational implications;
- elements relating to the conditions of the experiment (e.g. for a computer vision system: day/night, weather conditions, quality of the images used, ability to overcome any items blocking the view, etc.);
- elements relating to the potential risks of discrimination involved in the deployment of this specific AI system;
- elements relating to the implications of this system if deployed in an operational framework, taking into account the realities on the ground (for example, a false positive rate of 10% for 10 alerts does not have the same operational implications as 10% for 1,000 alerts).
Avoiding algorithmic discrimination
Issues
The use of AI systems can also lead to risks of discrimination. There are many reasons for this, with possible origins being:
- data used for learning, for example because it is non-representative or because, although representative of the “real world”, it nevertheless reflects a discriminatory nature (e.g. the reproduction of gender pay gaps); or
- the algorithm itself, which may contain design flaws. This aspect, also mentioned extensively in the European Commission's draft regulation, requires specific consideration by data controllers.
Examples
When monitoring an organisation implementing a system for the automatic assessment of video CVs recorded by candidates during a recruitment campaign, the CNIL noted the existence of a discriminatory bias. In this case, the system designed to qualify individuals’ social skills was not able to take into account the diversity of their accents.
The CNIL had the opportunity to assist the Defender of Rights in the publication of the report Algorithms: preventing the automation of discrimination. In particular, it calls for collective awareness and urges public authorities and stakeholders to take tangible and practical measures to prevent discrimination from being reproduced and amplified by these technologies.
Interested in contributing?
Write to ia[@]cnil.fr